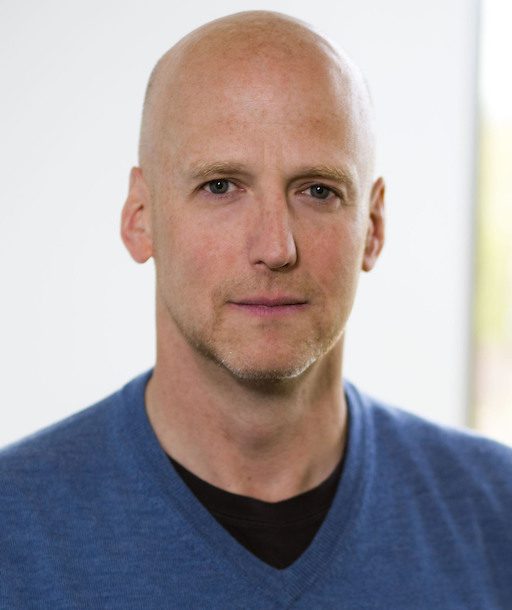
Gary M. Shiffman serves as the CEO of Giant Oak, Inc., and the CEO of Consilient, both of which are machine learning and artificial intelligence companies building solutions to support professionals in the fields of national security and financial crime.
Mission: Impossible
In the 1996 movie “Mission: Impossible,” Ethan Hunt (played by Tom Cruise) is part of an elite intelligence and national security team charged with safeguarding classified information. Hunt finds out some of his colleagues can’t be trusted, and thus begins one of the most successful movie franchises in history.
The summary, like most spy narratives, goes something like this:
-
People in positions of trust
-
perform missions to protect innocents, but
-
a betrayal happens.
-
The hero must overcome great challenges
-
for liberty and justice to prevail.
I call this the Ethan Hunt Problem, and people who work in national security, or seek to work in national security like the students I teach, rely on the real-life people of the Defense Counterintelligence Security Agency (DCSA) to make determinations of public trust. The professionals at DCSA have a uniquely important mission. They build the systems and adjudicate the ability and willingness of our colleagues in National Security to safeguard classified national security information. However, today’s DCSA professionals face the same challenge as all other risk professionals: data abundance.
More data exists in the world today than at any other time in history. This has created both opportunities and obstacles for security clearance reform and the DCSA. Many professionals I talk with believe that they have to make a choice in the face of so much data – either confine their search to smaller amounts of data or be overwhelmed by too much work for too few analysts, screeners, or investigators.
The “more data requires more people” narrative was true in the past. But now, thanks to behavioral science-based machine learning advances in entity resolution and topic modeling, more data can be used and less data overlooked when screening and vetting for risks at the same level of investment.
A Cautionary Tale
In approving $84 billion in fraudulent PPP loans, financial and government screeners both missed very obvious information by not using enough data. The concern at the time was that using too much data for screening PPP applicants would create too many false alarms, slowing the distribution of much-needed COVID-19 relief funds.
Earlier this year at Giant Oak, we did a study of the first 57 PPP loan fraud defendants charged by the DOJ for PPP fraud. When we ran these defendants through GOST, a machine-learning-based screening platform, we found that a full 25% would not have been approved had proper screening taken place.
The most common solution to the problem of sorting data was developed years ago by data-as-a-service providers, who create data sets that financial institutions and law enforcement agencies use to screen customers, applicants, and employees. These vendors create data sets by employing thousands of people to read news and arrest records in multiple languages and hand-tag this data to a name in a directory of people.
In addition to the privacy-invasive gag reflex one has when seeing these processes (large corporations collecting data on each of us), the hand curation comes at a high monetary cost. Think of thousands of people getting paid to read documents, sort them into piles someone they have never met might find important, and then discard the rest. Ethan Hunt might find this disappointing.
It is absurd that with the amount of data that exists today, we are still relying on data sets curated by humans and not algorithms. The data industry has been slow to adapt because it makes so much money; it is expensive, invasive, and imprecise – attributes our national security cannot tolerate.
As the banks that approved billions of dollars in fraudulent PPP loans have seen, the answer isn’t to use less data. The answer, instead, is to use machine learning to increase the data used for risk determination while simultaneously decreasing the number of false positives that would otherwise frustrate human screeners and investigators.
It is possible to investigate a large quantity of data without sacrificing quality. In fact, the opposite is true: by limiting the quantity of data, one also limits the quality of threat detection analytics.
Algorithms in GOST can reindex publicly available information on the internet (not just Google, the surface internet, but what is available on the deep web) for bespoke purposes and perform entity resolution on all that unstructured data to reduce the number of false positives. The result is efficiency with effectiveness. Security with privacy. More data without more work.
People on the front lines of national security and public safety and security face high stakes, and determinations of public trustworthiness require the highest degrees of effectiveness. Behavioral science-based machine learning finally enables screeners, analysts and investigators to do what they have always known to be the right thing: look across more data to find risk. The solution to the Ethan Hunt Problem is realizing that the data not used is the biggest risk of all.