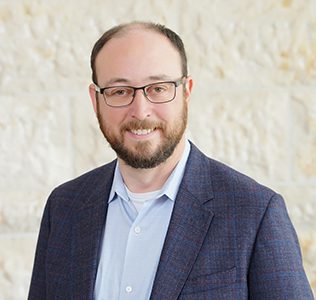
As the government contracting industry continues to partner within and with the federal government to augment human tasks with artificial intelligence, questions remain around current challenges, lingering opportunities and future implications.
And AI executives are working to answer those questions — because the technology isn’t new, and it’s being integrated in consumer and federal architectures.
WashingtonExec asked President and CEO of Reinventing Geospatial, Inc. Stephen Gillotte for insight on the nation’s AI strategy, competition, adoption and challenges. Gillotte established RGi in 2009 as a consulting and technology-driven business that works with the intelligence, federal, defense and aviation communities. RGi explores data science, geospatial data interoperability and network optimization, so he knows a thing or two about the emerging tech market.
What implications will the national AI strategy have on the federal government?
The U.S. national AI strategy has the potential of having a positive impact on the federal government, allowing us to optimize the services provided to our citizens.
I recall reading in Forbes Magazine that there are 2.5 quintillion bytes of data created each day, and that over the last two years alone, 90% of the data in the world was generated. In my opinion, your data is always trying to tell you something; you just need to learn how to listen.
If the federal government can harness this data through AI, and specifically machine learning techniques, then the government can provide optimized services for a lower cost, to and for our citizens. The prospects are nearly endless: fraud prevention, waste and abuse prevention, targeting resources to populations truly in need, improved intelligence, improved national security, etc., but all of this comes at a cost. There is obviously the privacy angle that needs to be considered.
In large masses, we have given up our privacy by choice to a number of corporations, such as Google, Apple and Facebook. On the national stage, do our citizens have the same choice of providing the federal government with this personal data? How does the federal government safely collect enough data on our citizens, communities and localities to support the AI and ML algorithms while maintaining citizens’ rights?
And alternatively, how willing, as a society, are we to provide the personal data required to optimize the delivery of these services? It’s a delicate balance the federal government — and industry — will no doubt struggle to maintain.
Which areas of government will have the largest implementation of AI?
The agency that harnesses and effectively manages the data is the one that is going to provide efficient services for citizens at an affordable cost. It could be the Internal Revenue Service with fraud detection, the National Institutes of Health with early health prevention mechanisms, or our intelligence community. But it ultimately will be those who are the best at capturing data, making it accessible through data standards, and exploiting the data.
What will be some of the common challenges as federal agencies begin adopting AI technologies?
Federal agencies will have to figure out the perfect teaming between people and AI; AI capabilities should be designed to leverage the human’s strength and leverage the machine’s strength.
AI algorithms, in addition to providing data and information in response to the data scientist’s question(s), need to provide the algorithmic evidence and reasoning supporting how the data and information was generated. Over time, a data scientist, armed with supporting evidence, has the opportunity to interpret and begin to trust the AI algorithm. Data scientists play their part on the team by learning how to interpret AI conclusions, which requires advanced education and training to understand the limitations of their AI teammates.
AI will enable a faster, more accurate and more thorough agency, but AI will require more work in the beginning, not less, as agencies begin adopting new data standards, tasking their staff with creating large numbers of examples for training machine learning models, and working with engineers to identify the opportunities with the highest impact.
A common challenge for many continues to be low-sample learning, or the ability to teach AI how to solve a problem without having many examples of the solution. Current approaches use thousands of examples to teach AI, whereas a person can often learn the same solution with just a few.
For federal agencies, especially those related to national security, low-sample learning is critical because many of the high-impact problems have few examples either because the events occur infrequently or staffing people to collect large numbers of examples is prohibitively expensive.
What are some of the considerations for GovCon firms that want to compete in the AI space?
The biggest consideration for GovCon firms is their ability to attract and retain top data science talent. There’s a war for talent. Commercial companies are quick to snatch up data scientists and machine learning experts. The number of candidates with both the expertise and the necessary security clearances is small, making recruiting difficult. GovCon firms need to be ready to retrain existing staff and work with colleges and universities to identify future AI leaders.
How will the business of AI evolve over the next years?
I saw in The Economist that “Data is the new oil,” and I couldn’t agree more. The renaissance in AI has been spurred by the availability of big data and the ability to process that data using machine learning techniques. Organizations are getting serious about AI and how they manage their data.
We will continue to see advances in new custom hardware, like Apple’s A11 Bionic chip and Google’s Edge TPU, designed to improve the speed, efficiency, and power consumption of deep learning algorithms. As this hardware moves into the cloud, it will contract the iteration cycle, drastically reducing the development time needed to create new AI solutions.