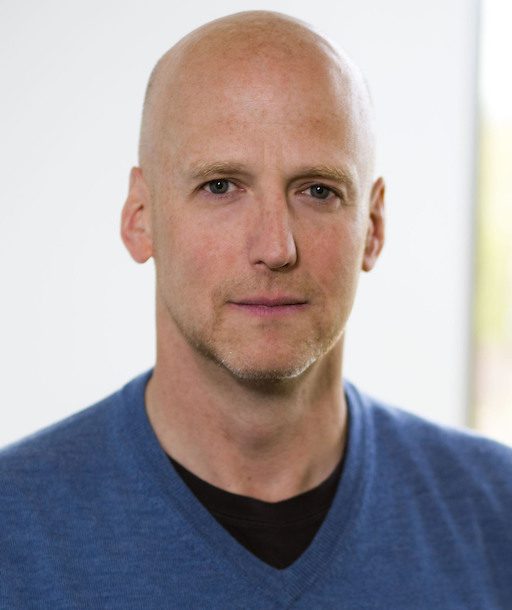
Gary Shiffman, the founder and CEO of Giant Oak, Inc., testified before the National Security, International Development and Monetary Policy Subcommittee on March 13, providing insight on how machine learning and artificial intelligence can help detect money laundering and illegal online trafficking.
The subcommittee falls under the Financial Services House Committee and held the hearing “Promoting corporate transparency: examining legislative proposals to detect and deter financial crime” to seek industry expertise and present considered legislation.
“Underground online trafficking now allows for simplified avenues to transport elicit materials across the nation and around the globe,” said subcommittee chair Rep. Emanuel Cleaver, D-Mo., at the hearing.
Cleaver’s opening remarks summarized concerns regarding the increase of financial crime, cyberattacks and technology attacks as they lead the worldwide threat assessment as a “paramount national security risk.”
There’s an increase in human and narcotics trafficking through online marketplaces, large-scale cyberattacks and money-laundering loopholes and a lack of financial transparency enabling criminals to falsify company status and facilitate terrorism and crime.
Shiffman, a professor at Georgetown University and a U.S. Navy Gulf War veteran, previously served as chief of staff at U.S. Customs and Border Protection. He has worked on anti-terrorism and homeland defense issues at an international law firm, and has advised senators as a national security and senior policy adviser to the U.S. Senate Leadership. He also has held various positions for the Defense Department — and was recognized as one of WashingtonExec’s Top AI Execs to Watch.
But Shiffman testified before the subcommittee as a technologist, he said, urging the nation must do better at combating these financial crimes, money laundering, trafficking and terrorism.
“Our current [anti-money laundering] regime requires radical reform,” Shiffman said at the hearing, citing United Nations statistics that show the amount of money laundered worldwide in one year is 2–5 percent of the global gross domestic product.
And though spending to combat money laundering and financial terrorism exceeds $7 billion nationwide, the Suspicious Activity Reports generated into the Treasury Department’s Financial Crimes Enforcement Network systems are mostly useless data. According to Shiffman, that data counters less than 1 percent of the problem — missing 99 percent of global financial crime.
To fix this, Shiffman recommends government and industry harness machine learning, AI and the application of behavioral science to data analytics, and focus them on supporting law enforcement and national security professionals.
These technologies can analyze, identify and find patterns in millions of rows of data the human eyes cannot. But to start, “to build the best machine to detect and deter financial crime, one needs good training data,” Shiffman said.
The best machines for anti-money laundering need to be trained on the best anti-money laundering data, and applying these systems to financial crimes is where the opportunities lie.
Government agencies know which Suspicious Activity Reports provide the best quality data, but banks don’t, so they can’t train the tools properly, Shiffman said. In fact, the few banks that use machine learning for anti-money laundering train the machines on old Suspicious Activity Reports.
“If more than 95 percent of past [Suspicious Activity Reports] were wrong, then these banks simply perpetuate inaccuracies,” Shiffman said. But with feedback from law enforcement and national security professionals, the system can improve with better data. This is the direction anti-money laundering and AI needs to go in.
Shiffman also provided three core principles the subcommittee should consider as part of reform or legislative proposals:
- Encourage information sharing between law enforcement, financial institutions and regulators for better training data quality.
- Avoid solutions that lack transparency and make it more difficult for humans to understand internal processes or outcomes of the machines.
- Humans should still be judging what is good and bad or right and wrong. Let machines sort and filter data.
These recommendations come as the subcommittee is considering three acts: the Kleptocracy Asset Recovery Rewards Act, the Corporate Transparency Act of 2019 and a bill to make reforms to the Federal Bank Secrecy Act.
The kleptocracy bill would establish a program within the Treasury Department to reward people providing information that leads to the restraining, seizing or forfeiture of stolen assets linked to government corruption.
The Corporate Transparency Act would require companies in the U.S. to disclose financial beneficiaries and owners to the Financial Crimes Enforcement Network, to prevent criminals from exploring company status for criminal gain. Currently, there is no law doing so, meaning money launderers are using anonymous shell companies to hide their money for illegal activity.
The reform to strengthen the Federal Bank Secrecy Act and anti-money laundering laws will update legislation to include the current and advanced threat environment, providing financial institutions with more guidance on where to focus anti-money laundering program efforts and innovation.
Visit Giant Oak’s website to review Shiffman’s full remarks.