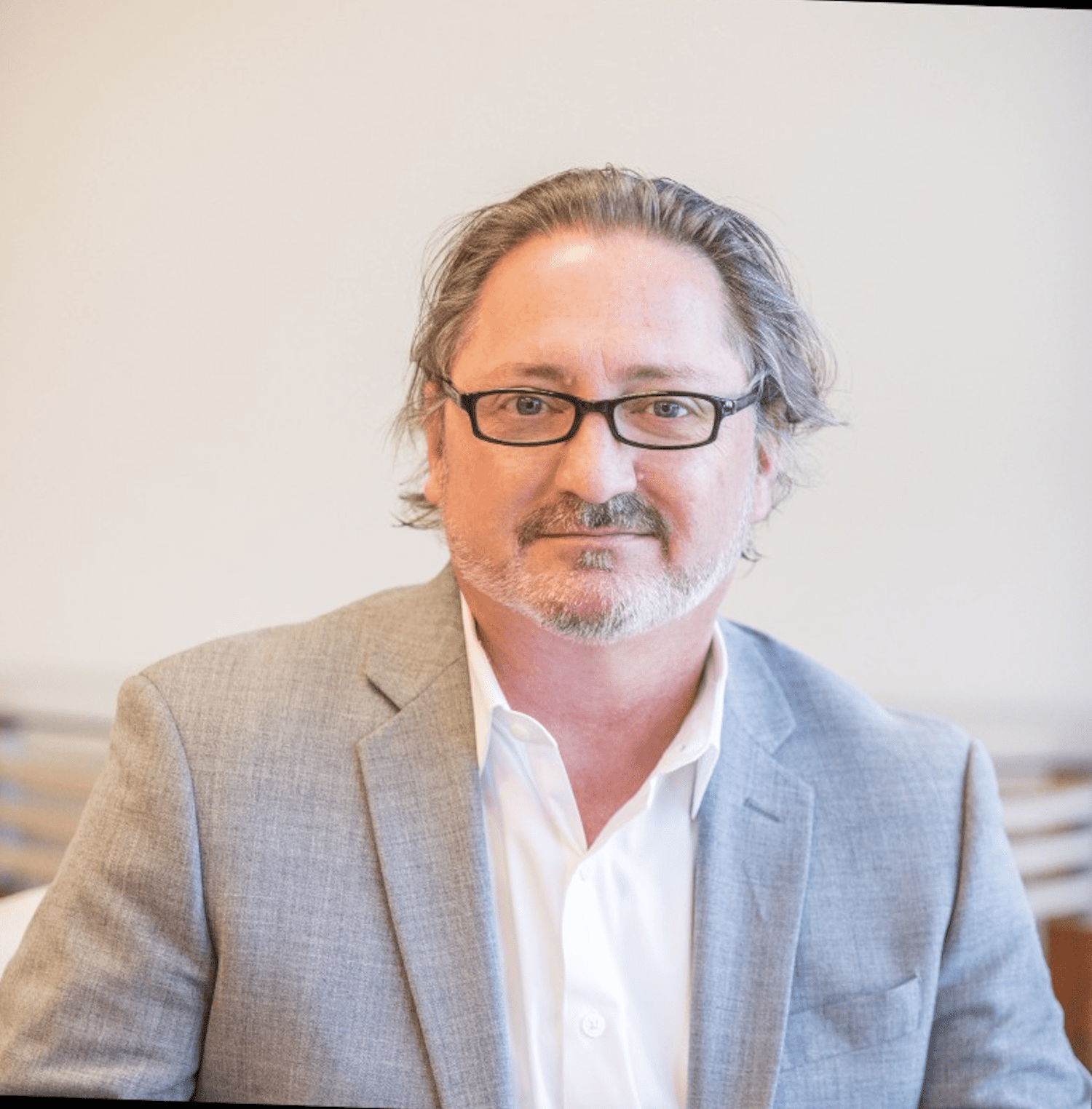
The finalists for WashingtonExec’s 2024 Pinnacle Awards were announced Sept. 20, and we’ll be highlighting some of them until the event takes place live, in-person Nov. 21.
Next is Mark Poe, chief scientist, data science & analytics at Core4ce, and finalist in the Healthcare Executive of the Year, Private Company, category. Here, he talks about recent achievements, shares career advice and more.
What key achievements did you have in 2024?
I’ve been very focused on the application of advanced AI/ML technologies to enhance the reliability of open-source intelligence and provide a powerful framework for early detection and response to public health threats. Since developing and deploying Project Bioscape in 2024, a bespoke subscription-based biosurveillance data feed, I have continued to optimize its performance. The foundation of this feed is built on a data analysis tool that utilizes AI and machine learning (ML) to correlate and analyze diverse data sets, called the Intelligence Correlation Engine and the Corroboration Index.
Recently, I have enhanced Project Bioscape’s ability to deliver timely and high-quality data insights to prioritize critical information sharing by integrating OSINT with data from disease vectors, news reports, and scientific journals to create a real-time surveillance system for detecting emerging public health threats. Together, ICE and the Corroboration Index have already significantly enhanced the early detection of public health threats, ensuring decision-makers receive the most accurate threat detection and assessment information.
What are your primary focus areas going forward, and why are those so important to the mission?
A few years ago, I was inspired by “The Book of Why” by Judea Pearl. In it, Pearl describes a monumental shift in the way we think about statistical reasoning, moving from correlation to causation. My professional focus ever since has been on causal inference and Bayesian networks because I believe they are fundamental to the future of AI. Combined, they enable a shift from correlation-based pattern recognition to true causal understanding and decision-making under uncertainty.
As AI systems move into high-stakes domains like healthcare, the ability to distinguish cause from correlation becomes essential for reliable, actionable insights. Causal models empower AI to reason about interventions and “what if” scenarios, allowing for counterfactual reasoning that enhances fairness, trustworthiness, and accountability. Bayesian networks further strengthen AI’s ability to handle uncertainty, enabling more adaptable, probabilistic decision-making in real-time. Together, these techniques provide a path toward more transparent, interpretable, and resilient AI systems that not only predict – but explain and optimize – outcomes across diverse, evolving environments.
What is your best career advice for those who want to follow in your footsteps?
Never stop learning. I like to say that if you’re the smartest person in the room, you’re probably in the wrong room.
Fun Fact: What is something about you that most people do not know?
I enjoy destination hiking and have completed several all over the world: Hadrian’s Wall in Northern England (82 miles), the Southwest Coast Path in Cornwall (54 miles), the Great Glen Way in Scotland (70 miles), the Malerweg Trail in Germany (72 miles), among others.