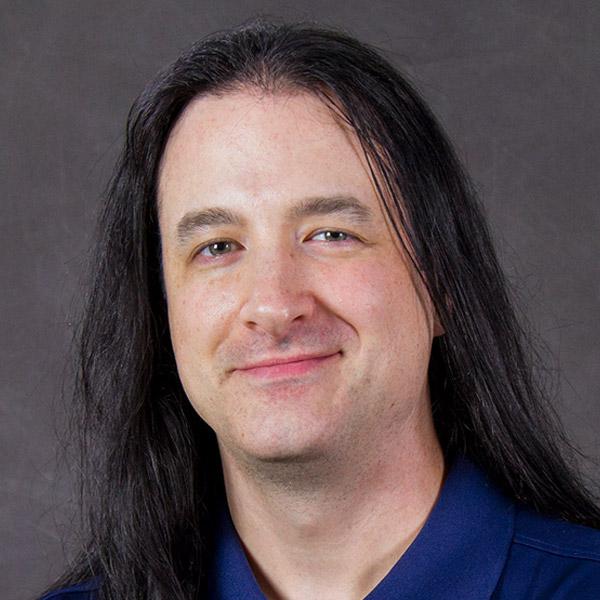
Aaron Dant, ASRC Federal
Aaron Dant is ASRC Federal’s chief data scientist and architect, designing cloud scale analytic environments using machine learning, Natural Language Processing, and human behavioral analysis capabilities. He’s a technology evangelist for solicitations and business development, training staff, expanding the artificial intelligence service offerings and conducting principal research. Dant most recently spent nearly 15 years leading analytic programs, including: signals processing, human language technology, geospatial, cellular, medical, and human cultural and social behaviors. Dant’s main focus has been the software engineering world, designing and executing large-scale enterprise production systems.
Why Watch:
Dant believes it’s crucial to share thought leadership as customers try to make sense of how they can best leverage AI and machine learning solutions to support their missions. He advocates for the secure and ethical use of AI tools and platforms, and he contributes to thought leadership in production of ML implementations, fraud/waste/abuse detection, predictive analysis, informational warfare and augmented search. Dant is also a sought-after speaker and a core researcher of computer augmented informational warfare. He has co-authored three peer-reviewed papers on this topic this year, including “This One Simple Trick Disrupts Digital Communities.”
“My recent papers are related to identifying radicalization and adversarial influence operations in online communities, which is really pertinent right now,” Dant said.
Working with federal data, it’s often tough to apply deep neural networks as the volume and distribution of the available tagged data can be scarce. His team depends on simulation to generate massive amounts of representative tagged data to build its models. As the model is applied to real data over time, the simulations get updated in a roundtrip to better match the real-world conditions.
When it comes to specific technologies, Dant focuses on Java, Scala, Python, R and big data technologies such as HBase, Accumulo, Spark and MapReduce. Some of the AI solutions he’s helped develop are created as an a la cart selection of MicroServices and analytic tools running on Amazon Web Services with an Angular front-end.
Looking at the near-term challenges with AI, Dant points to the fragility of some of the technologies.
“ML techniques are extremely effective at certain tasks, but have proven to be brittle, and in the case of deep networks are difficult to provide transparency for why a particular decision was made,” he said. “This results in ‘black box’ systems, which are potentially dangerous. Additionally, all ML models are only as good as the data they are trained with, presenting potential unintended consequences of bad models trained on insufficiently diverse data.”
While government and industry alike face challenges across the field, “the federal space is more at risk as there are fewer pipelines to sufficiently label large and diverse data sets,” Dant said. “So, ensuring AI tools are both ethical and secure is ASRC Federal’s primary objective when designing these systems.”
Beyond the challenges with AI, technology writ large moves through a phase of “barely manageable” systems, where numerous individual systems or agents interact in diverse but defined ways, and into a place where complex systems begin to have unplanned behaviors as the services, devices and AIs form an autocatalytic ecosystem, Dant said.
“I genuinely believe this is going to require a paradigm shift in how software is designed going forward taking cues from multidisciplinary research including ecology and information science,” he said.
2 Comments
Pingback: Who’s Winning the Global Battle for AI Leadership – Netmetic
Pingback: Who’s Winning the Global Battle for AI Leadership - Data Science Tidings