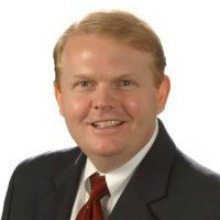
After attending NVIDIA’s GPU event in November, Primehook Technology CEO and Founder Leif Ulstrup reflected on the conference’s prominent topic: deep learning technology. Ulstrup found discussions at the conference did not touch on how this technology will impact management, something worth consideration before adopting the new technology.
WashingtonExec had the opportunity to chat with Ulstrup further about the future of deep learning technology.
WashingtonExec: What are some of the benefits to adopting deep learning technology?
Leif Ulstrup: We are on the cusp of the newest revolution in the application of computers in business and government. Investment is shifting from streamlining workflows to new applications that augment human interpretation and judgment.
The next wave in productivity improvement will be applications that diagnose situations. Automation will arrive in fields we once thought were the exclusive domain of human experts. These systems will complement knowledge workers.
The early applications will be narrow in scope but broad in utility. For example, automated sentiment analysis of text from internet comments is already assisting customer service representatives. Deep learning technology, a type of machine learning, is powering this revolution.
WashingtonExec: What’s the biggest difference between deep learning and current machine learning methods?
Leif Ulstrup: Deep learning is a type of machine learning. It has generated lots of press buzz. The excitement is due to the major advances it has enabled in a very short time. Computer vision and natural language processing are two of the toughest topics in artificial intelligence research. Progress in those fields was advancing at a very slow pace.
In 2012, the winning entry in an annual computer vision competition incorporated deep learning. The winner showed major improvements over traditional programming approaches. This sparked interest in using deep learning for a broad set of problems. Innovations in deep learning technology and applications have exploded since then.
Machine learning applications use a different approach than conventional application programming. In a traditional approach, an analyst studies the problem to uncover patterns and rules. The analyst then documents how the computer should respond to inputs.
Next, a programmer converts the specification into a program. This approach works well for situations where all the input conditions are known and the rules represent a well-documented policy. If the data are complex or noisy and the rules are not easy to specify because they depend on human judgment, traditional programming does not work very well. That’s where machine learning shines.
In machine learning, the analyst/programmer thinks of the problem as a learning model selection and training task. Machine learning algorithms use traditional programming to specify how the learning model works. The difference is that the rules the learning model develops comes from “training” the model on example input/output patterns.
The model makes small adjustments to its internal representation as it cycles through training data. The model training stops when the prediction accuracy reaches a plateau. The programming defines the architecture of the model and how the model adjusts to new ‘training’ patterns.
The analyst needs to be good at selecting the appropriate machine learning models and the training data. The modeler may have very limited expertise in the application domain though.
Since 2012, the pace of innovation in simplifying deep learning tools is astonishing. Deep learning models and techniques that took months to program a few years back can today be set up with a few lines of computer code. The models and techniques are available to anybody with basic computer scripting know-how. They are no longer limited to academic AI researchers.
For instance, in medicine this year’s Radiological Society of North American conference agenda is full of deep learning talks. Deep learning applications in radiology will likely revolutionize the field across the globe. Access to care will become more abundant. It will enable experts to focus more of their time on the toughest cases.
WashingtonExec: While the RSNA conference focuses on the application of deep learning to medicine, do you think it can/will be applied in other industries?
Leif Ulstrup: The enthusiasm in medicine about the potential of deep learning should be a wake-up call in other fields. Medicine represents some of the most complex human judgment and diagnostic problems. The pace of innovation and application in medicine is even surprising to those in the field.
A January 2017 Stanford publication “Deep learning algorithm does as well as dermatologists in identifying skin cancer” illustrates the power of these advances in matching the diagnostic ability of the best humans. Similar results are emerging in pathology and radiology. If this technology can revolutionize medicine, it will have an even broader impact in business and government.
Incremental improvements that complement the knowledge worker are the place to start experimenting. Many professionals spend considerable time on low value-added activities surrounding their core expertise. The first experimental applications of machine learning should focus on reducing those burdens. An easy place to start is to look for workflows where a human is spending time segmenting incoming cases before the real knowledge work begins.
WashingtonExec: You note this topic was one that dominated the conference and that the presentations were “crowding out other topics at large international medical conferences.” What topics do you think should be covered more?
Leif Ulstrup: The biggest challenge with a technology that is so disruptive will be in adoption. For individuals, deep learning advances are likely to change how professionals think about their career path. For large organizations, deep learning is likely to disrupt their economics and social fabric. Leaders in those organizations will need to understand the technology, upgrade strategies, revise systems and prepare their people to adapt.
For regulated industries, there will be enormous tension between the innovators and regulators. The topics in future conferences will likely revolve around navigating those challenges.
WashingtonExec: Are there any technology trends you think should be discussed more at conferences such as this?
Leif Ulstrup: There is a never-ending stream of new ideas in the information technology field. That’s what makes it so much fun. I have no doubt there will be many new innovations that emerge as a byproduct of the success of deep learning.
For instance, as the cost of interpreting medical imaging declines and the quality goes up there will likely be more innovation around low-cost, portable imaging technology. That might replace other types of medical diagnostics and cause further disruption to adjacent fields.
The biggest challenges are likely to be preparing our children and workforce to be lifelong learners. Embracing innovation and adaptation have always been the hallmark of successful organizations. Success depends on the quality of the talent and the vision of the leadership team.
As the world of work becomes more digital, the potential for disruptive innovation grows. My advice for professionals is to prepare for these advances with continuing education. My advice to senior executives is to hire talent that embraces innovation and start experimenting with the deep learning before a competitor catches you off-guard.