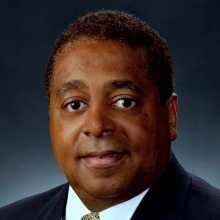
Data science — it’s what provides organizations the world over with the strategic edge they need to stay competitive or deliver enhanced citizen services. But what does it entail? Why is it so important right now? And most importantly, where is it going next?
WashingtonExec sat down with Melvin Greer, the director of data science and analytics at Intel Corp., to get some answers. Here’s what he told us:
The latest advances in data science are driven by the convergence of three things. First, data is the fuel that drives innovation in federal government and in commercial organizations. Big data is a critical thing. Second, we have better, more affordable computation processing capabilities. In 1956, when the term artificial intelligence was coined, scientists were just experimenting with direct-input keyboards. Finally, we now have better algorithms. The combination of big data, increased computational power using advanced algorithms is generating innovations that are transforming government and commercial organizations.
The taxonomy for data science includes a number of terms which require some granular definition: core analytics; cognitive computing; machine learning, deep learning and neural networks; and then ultimately, artificial intelligence.
Core analytics are the four analytic capabilities that have been born out of the discussion of business intelligence, namely descriptive, diagnostic, predictive and prescriptive analytics. This is essentially what happened, why did it happen, what is likely to happen next, and then, what should I be doing about it so that I can take advantage of it?
With cognitive computing and machine learning, we are working to extract patterns or features from data in order to solve predictive problems. These patterns rely on algorithms and programs that are generally designed to learn from past experiences. That learning process involves calibrating or training the system, and testing it through inference. We teach it in much the same way we would teach a child. And just like a child, eventually, the system is able to learn on its own.
Deep learning and neural networks are really a subset of machine learning. Deep learning tends to model a set of high-level extractions based on layers of analysis. These layers can be interconnected, forming a network like a neural net in biology. These neural networks have many filters in the layers, and each layer adds more and more detail so that you can get a sense of what’s going on and derive insight.
Ultimately, we have artificial intelligence. While there are many people floating definitions out there, there’s really not a solid definition that all practitioners adhere to. There are five key characteristics of artificial intelligence.
1) Logical reasoning
2) Knowledge representation — the ability to take a single piece of information and apply it to multiple contexts, or to derive new information and knowledge from disparate known information
3) Planning and navigation
4) Natural language processing
5) Perception and awareness
All of these terms fall under “data science,” but it’s important to differentiate them because practitioners don’t use the same tools to do the same things. For example, autonomous vehicles leverage deep learning, which is different from many cybersecurity tools that leverage predictive analytics to extrapolate patterns and identify potential threats.
The challenges facing the nation will ultimately require all of these tools — and more —making now the best time to be working in data science.