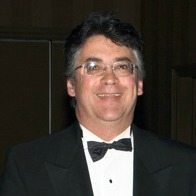
Technology executive Louis Chabot has over 25 years of experience working with information systems. As the big data lead at DRC, he used his background in the financial sector to work on a cloud system for government clients. He attributes success at the professional service delivery company to understanding customer needs, and putting them first.
In this interview, Chabot names the six dimensions of big data as he sees it, as well as the multideminsional big data problems the federal government is now facing.
WashingtonExec: You have over 25 years of experience in information systems. Can you expand on your background and tell me how you got into the role that you are in now?
Louis Chabot: In the past, I worked on Wall Street systems that generate extremely huge amounts of data. If you think of trading systems huge amounts of data are created and exchanged every second. I transitioned my knowledge of the commercial financial markets to the government sector and I became responsible for developing systems for the government that also include large amounts of data. For the last four years I’ve been working on a very large cloud project where we take over 400 different data sources and through various techniques we merge these at together in a big data capability to give the analysts some pretty powerful search, analysis and visualization capabilities.
——————————————————————–
“DRC looks at big data problems in six dimensions; volume, variety, velocity, variability, visibility and value. This last dimension, value, is particularly critical.”
———————————————————————-
WashingtonExec: Do we have too much data? Is this causing us to miss things?
Louis Chabot: I would say too much useless data but not too much valuable data! DRC looks at big data problems in six dimensions; volume, variety, velocity, variability, visibility and value. This last dimension, value, is particularly critical. The value dimension of big data helps us to understand whether data truly needs to be captured, processed and stored at a given time or if it should be processed later. Proper consideration of this value dimension can reduce data volume and help make an otherwise complicated problem much more feasible. At DRC we refer to these kinds of decisions as a big data roadmap. Typically organizations have a good roadmap for operational data but have a very poor or nonexisting roadmap for decision support data. Without a roadmap you may accumulate the wrong data or have essentially useless data but not accumulate the data that is really valuable.
WashingtonExec: What would you say is the biggest data problem we are facing right now?
Louis Chabot: The issue with data is multidimensional. The first is the ability to understand providence. Providence is who, when and under what circumstances data was created – so that pedigree can be understood. Pedigree is a decision such as trustworthiness of data that can be made when it’s time to use the data. A lot of data that we have today does not come with providence information or people do not use that provenance information to make pedigree decisions. Therefore they blindly trust the data without considering providence or pedigree. The second issue with big data is what I call the lack of upfront planning or architecture. Big data problems are by definition complex problems. They are not something you can design on the back of a napkin. These are extremely complicated problems and I think there is insufficient upfront planning or architecture. Another aspect is that big data software is particularly difficult to develop from a variety of perspectives. When you develop big data solutions, you have to take into consideration the functional requirements such as the business rules but also a variety of non-functional requirements; those are things like scalability, performance and reliability. The intersection of functional requirements and non-functional requirements makes these kinds of problems extremely difficult to solve. All of these issues essentially can be addressed by having what we call a big data reference architecture that is then used as a framework for these complex problems. The key is they need to be architected from the front.
——————————————————————-
“Typically organizations have a good roadmap for operational data but have a very poor or nonexisting roadmap for decision support data. Without a roadmap you may accumulate the wrong data or have essentially useless data but not accumulate the data that is really valuable.”
——————————————————————
WashingtonExec: How do you think the increase in data mining/analysis will change federal contracting?
Louis Chabot: Today it is particularly difficult to define upfront good requirements for data mining or analysis. If you can’t come up with good requirements then you can’t come up with good completion and acceptance criteria – this is going to affect the way you write contracts for procurement. For example, you may have to change clauses around warranties on the produced software and you may change how the work is conducted. In this case you would need more government participation to define the requirements and validate the software. The second point is around new source models for these kinds of software. Traditionally, software was custom developed in house or it was procured through some COTS (commercial off-the-shelf) or some GOTS (government off-the-shelf) and was integrated. You are seeing analytics as a service as an evolving delivery model. That is essentially part of an overall trend towards cloud services that is going to affect contracting. My last point is increasing dependencies on other agencies. Big data solutions require joint participation or involvement from a lot of various agencies. For example, data sourced by another agency, or on a cloud infrastructure supplied by another agency. Dependencies to a contractor essentially mean risk and these different operating conditions need to be accounted for in the procurement process.
WashingtonExec: Why do you think it is important to archive unstructured data like social media trends?
Louis Chabot: It’s important to understand what the value of social media data today and tomorrow is and then use this value assessment as the filter for maintaining this data or archiving it. We also need to consider the timeliness of social media. Social media generally has a relatively short time horizon; sometimes just from a few hours to a few months. This kind of data, because of its short time horizon, can be easily summarized and aggregated to support longer term historical trend analysis without requiring the storage or archiving of the large raw data.
WashingtonExec: What is the largest roadblock you’ve run into when rolling out large or small data mining or analytic programs?
Louis Chabot: I think there are four important criteria. The first one is expectation mismatch. The bottom line is that analytics are just software. There is nothing magical about them except that they can be very complex, so from the outset there is an expectation mismatch. The next thing is analytics software development challenges – analytics are a special kind of software. Analytics are very complex from the functional and nonfunctional perspective as I mentioned earlier. Writing these kinds of software requires pretty advanced software engineering, usually statistical, sometimes requiring some scientific or business domain experience. That skill intersection is very difficult to get. Testing analytics are super difficult. From a functional perspective it is difficult to determine if the rules are correct and from a nonfunctional perspective challenges arise for things such as performance and scalability. All of these roadblocks that we talked about – expectations mismatch, software development, not planning upfront correctly, and testing – the tricks of building analytics and the tricks of validating analytics all together at DRC we tackle them through a package we call a “Big Data Analytical Roadmap.”
WashingtonExec: As the contracting community prepares for cuts in federal spending why do you see big data as an opportunity for growth?
Louis Chabot: From the government’s perspective (or the demand side) there’s two issues here; an offensive and the defensive posture. First from an offensive posture. Whether you are tracking down terrorists or criminals or you are monitoring legitimate activities domestically or abroad, complete and up to date data is critical for the federal government to operate in such an environment. This is going to require continued investment in big data solutions. Nobody wants, for example, another 9/11 on their watch. Now from a defensive posture, with a reduction in budgets, the government is going to have to look for efficiencies in their processes. Sharing of data across agencies to remove duplication is another type of efficiency that the government will be looking at. Finally sharing data such as the data.gov open data initiative will increase government transparency and essentially lead to better assessments of program or policy effectiveness.
Now let’s look at it from the supply side. Big data is not something you can just start selling tomorrow. It takes years of experience. You need to have data domain knowledge, business domain knowledge and complex system experience. Service providers that have these capabilities will have plenty of work.
WashingtonExec: What is the best piece of business advice that you have received?
Louis Chabot: I used to work for a product vendor and one day I decided I was going to hang up my own shingle and essentially call it Louis’ Consulting Shop. I needed to figure out what the critical success factors of running a professional service delivery company were. Essentially it is to be true to your customer and put their interests ahead of yours. So essentially understand what drives them, what are their priorities but also understand what are their preferences as well as any constraints or limitations they may have. Taking all of that together permits me to offer a solution that is maybe not perfect for me as the supplier, but is certainly better for them as the consumer or purchaser of that solution.
WashingtonExec: What keeps you up at night?
Louis Chabot: Let’s roll back about five years ago when the technology market changed about every 12 to 18 months. I knew if I watched Oracle and IBM and the SAS’s of the world, and I followed them about every 18 months, that I could stay ahead of it. The technology markets didn’t change that much. Now it’s completely different. There are literally thousands of sources of technology solutions and the open source software market is one of them. A lot of these sources of solutions are not traditional companies—some of them are academic, some are a couple of guys in a garage—and they are changing not every 12 to 18 months, but every 6 to 8 weeks. Now every 2 or 3 months I find myself having to shift technology curves with a much larger number of solution candidates. My customers expect me to stay on top of these; unfortunately it is extremely difficult because of the speed and the volume of technical solutions that are now available. At night when I go to bed and close my eyes I try to figure out on which portion of the technology curve to focus on.